Advanced Cohort Analysis Techniques for SaaS Growth: Tracking, Segmenting, and Predicting Churn and Retention
In the competitive landscape of Software as a Service (SaaS), understanding customer behavior is crucial to achieving sustainable growth. Cohort analysis allows SaaS companies to track customer retention, identify at-risk users, and predict churn with precision. Advanced cohort analysis goes beyond basic metrics, leveraging sophisticated segmentation and predictive machine learning models. This article delves into technical cohort analysis strategies that optimize tracking, segmentation, and churn prediction for SaaS growth.
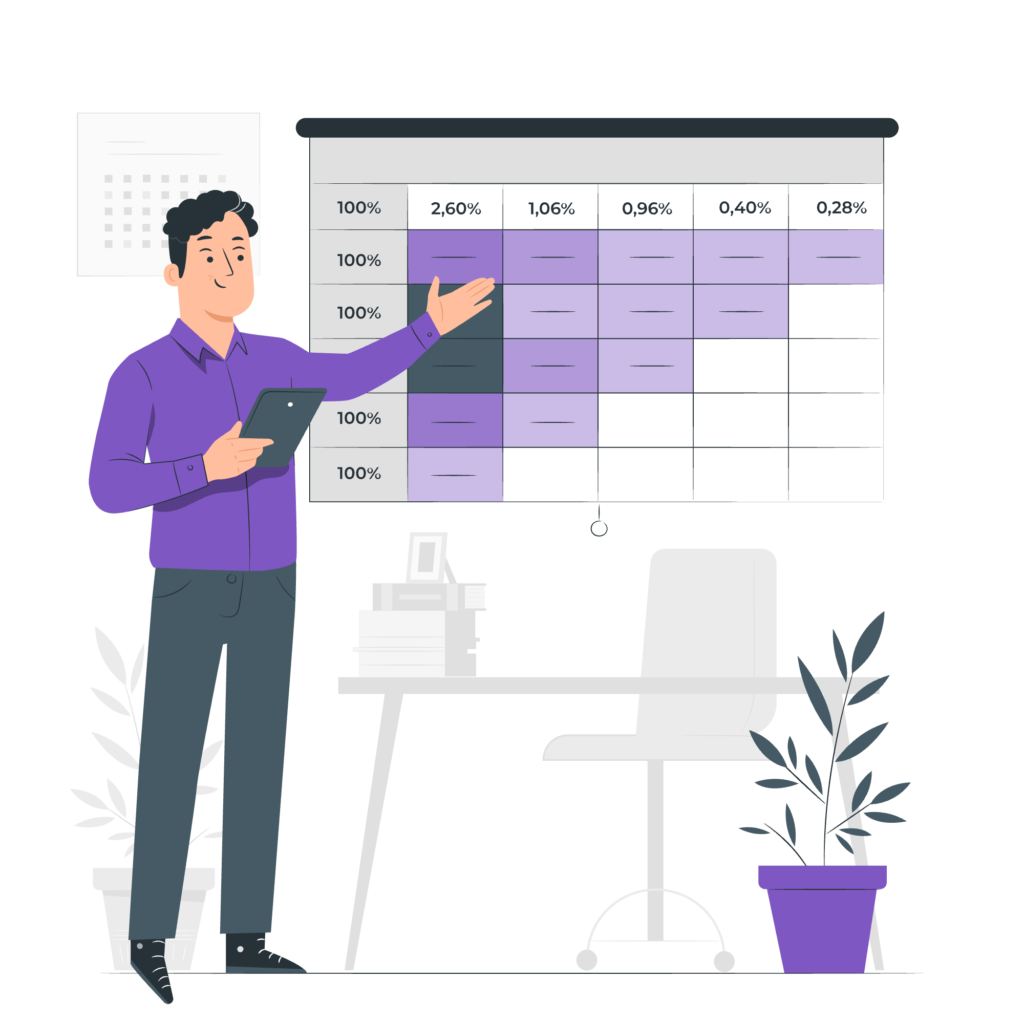
1. Understanding Cohort Analysis in SaaS
Cohort analysis involves grouping users based on shared characteristics, typically by the time of acquisition or certain behavioral actions, to observe their behavior over time. In SaaS, cohort analysis often focuses on customer retention and churn trends to gauge long-term growth potential. Traditional approaches include time-based and behavioral-based segmentation, but advanced techniques leverage machine learning and predictive analytics to gain actionable insights.
Key Benefits of Cohort Analysis for SaaS:
- Retention Tracking: Identifies high-retention cohorts to tailor strategies that foster loyalty.
- Churn Prediction: Anticipates users likely to churn, enabling preemptive actions to retain them.
- Lifecycle Analysis: Examines user journeys and lifecycle stages, refining acquisition and engagement strategies.
2. Advanced Tracking Techniques for Cohort Analysis
a) Event-Driven Tracking for Behavioral Cohorts
Event-driven tracking focuses on user behaviors, categorizing cohorts by specific interactions with the software. This approach is crucial for identifying feature usage patterns, which are often predictive of retention and churn.
Implementation Steps:
- Define Key Events: Identify the critical actions (e.g., account creation, subscription upgrade, feature usage) that correlate with user engagement and retention.
- Set Up Event Tracking: Use analytics platforms (e.g., Mixpanel, Amplitude) to track these events in real-time.
- Analyze Event Frequency: Segment cohorts based on the frequency and depth of feature engagement to pinpoint behaviors associated with loyalty or churn.
b) Time-Based Tracking for Lifecycle Analysis
Time-based tracking allows you to evaluate cohorts by their journey stages and lifecycle moments, such as onboarding, renewal, or trial expiration. Tracking cohorts through these stages enables a deep dive into user retention patterns at various points.
Example Technique:
- Track users who signed up within a specific timeframe and observe their retention rates month-over-month.
- Use this data to refine lifecycle marketing strategies, such as targeted renewal campaigns or personalized in-app messaging during critical decision points.
3. Segmentation Techniques for SaaS Retention Optimization
Segmentation in cohort analysis empowers SaaS companies to understand user variability and customize strategies for different customer segments. By diving into demographic, behavioral, and psychographic characteristics, you can improve retention through targeted interventions.
a) Demographic Segmentation for Personalized Messaging
This technique segments users based on characteristics like industry, company size, or location. It’s especially useful for B2B SaaS, where customer needs vary significantly by these attributes.
Steps for Demographic Segmentation:
- Collect Data: Use signup forms and onboarding processes to gather demographic details.
- Cluster by Demographics: Group cohorts with similar demographics and observe their churn and retention rates.
- Customize Outreach: Tailor in-app messaging and email campaigns to each demographic cluster, aligning content with industry needs or business size.
b) Behavioral Segmentation Using RFM (Recency, Frequency, Monetary Value) Analysis
RFM analysis segments users based on their recent activity, engagement frequency, and spending. Though traditionally applied in e-commerce, it’s highly effective for SaaS retention, providing insights into high-value users and identifying those at risk of churn.
Implementation of RFM Segmentation:
- Recency: Track the last interaction date, highlighting recent activity or inactivity.
- Frequency: Measure the frequency of key actions within a time frame (e.g., weekly logins).
- Monetary Value: Consider the value of active plans or upgrades, identifying high-value cohorts.
Combine RFM scoring with cohort analysis to pinpoint customers with high engagement and revenue potential and to create tailored upselling strategies.
c) Psychographic Segmentation for Engagement Enhancement
Psychographic segmentation involves categorizing cohorts by their motivations, interests, and behavior patterns. This approach requires qualitative data, often gathered through surveys or in-app feedback mechanisms.
Practical Application:
- Use psychographic data to develop customer personas.
- Tailor product features and messaging based on user motivations, such as productivity-focused or collaboration-focused users.
- Drive higher retention by refining features and content around these user needs.
4. Predicting Churn with Machine Learning Models
Predicting churn with machine learning goes beyond traditional regression models, using advanced techniques to account for complex user behaviors. Machine learning models can forecast churn probabilities and identify which cohorts are most at risk. Common approaches include logistic regression, decision trees, and neural networks.
a) Logistic Regression for Churn Prediction
Logistic regression is often the starting point for churn prediction. It evaluates how various independent variables (e.g., feature usage, time since signup) affect the likelihood of churn.
Steps:
- Feature Selection: Identify key predictors such as login frequency, support tickets, and usage duration.
- Train the Model: Use historical cohort data to train a logistic regression model.
- Analyze Results: Identify patterns that most influence churn, such as users with low monthly engagement.
b) Random Forests and Decision Trees for Enhanced Accuracy
Decision trees and random forests improve on logistic regression by creating multiple decision paths, which are ideal for capturing non-linear patterns in user data. Random forests, which aggregate multiple trees, help increase prediction accuracy and robustness.
Benefits:
- High Precision: Random forests reduce the risk of overfitting, common in simpler models.
- Actionable Insights: The tree structure helps identify specific feature combinations or behaviors associated with churn.
c) Neural Networks for Complex Cohort Predictions
Neural networks offer high-level predictive capabilities for more nuanced churn predictions, although they require larger datasets and computational resources. They are ideal for analyzing a vast number of variables, capturing interactions that simpler models may miss.
Steps to Implement:
- Data Preprocessing: Normalize and preprocess your cohort data to feed into the neural network.
- Model Training: Train the model on historical churn data, iterating to optimize prediction accuracy.
- Deploy and Iterate: Use predictions to target at-risk cohorts with retention strategies, refining the model over time.
d) Survival Analysis for Time-to-Churn Predictions
Survival analysis estimates the duration until a customer churns, rather than just predicting whether they will churn. This technique provides a timeline view, enabling SaaS companies to implement retention tactics at key stages.
5. Leveraging Cohort Analysis Insights for Retention Strategies
Cohort analysis findings should inform actionable retention strategies across the customer lifecycle. Effective implementation of insights from advanced cohort analysis requires cross-functional alignment between marketing, product, and customer success teams.
a) Targeted Re-Engagement Campaigns for At-Risk Cohorts
Use predictive analytics to identify at-risk cohorts and deploy re-engagement campaigns. Tailored content such as educational resources, product tours, or promotional discounts can entice users back into the platform.
Key Tactics:
- Dynamic In-App Messages: Engage users during login or product interaction with personalized reminders and tips.
- Email Drip Campaigns: Automate email sequences that highlight key features or offer time-limited incentives.
b) Feature Adoption Programs for High-Retention Cohorts
For cohorts demonstrating consistent engagement, foster loyalty through targeted feature adoption programs. These programs encourage deeper usage of high-retention features, reducing the likelihood of churn.
Examples:
- Onboarding Enhancements: Personalize onboarding experiences to each user type, promoting features most relevant to their needs.
- In-App Education: Provide in-app walkthroughs and tooltips to guide users through underutilized features.
c) Feedback-Driven Product Development for Retention
Feedback from cohort analysis should directly inform product development. By understanding which features are used by loyal customers, SaaS companies can refine the product roadmap to prioritize high-retention attributes.
Conclusion
Advanced cohort analysis techniques enable SaaS companies to drive growth by refining their approach to customer retention and churn prediction. By tracking event-driven and time-based behaviors, implementing targeted segmentation, and deploying machine learning models, SaaS companies can achieve a nuanced understanding of customer needs. Predicting churn with precision and implementing insights across teams empower SaaS providers to enhance user engagement, minimize churn, and create a scalable growth path.
Mastering these advanced techniques is critical in the highly competitive SaaS environment, where retention and growth are inextricably linked.